Violent extremism is still spreading online. There’s a way to stop it.
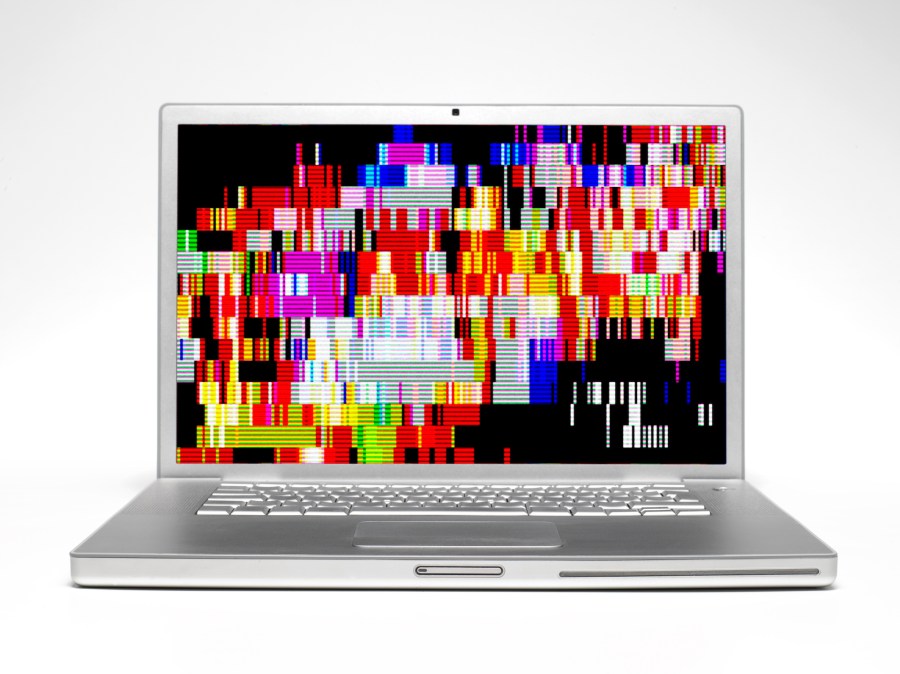
Buffalo, El Paso, Glendale, Ariz., and Colorado Springs. Those are just a few of the cities that have been racked by social media-fueled terrorist attacks since 2019. Statistically, it will only be a matter of months before the U.S. witnesses another terrorist attack committed by a “keyboard warrior.”
It has been four years since a lone gunman live-streamed his murder of 51 people at two mosques in Christchurch, New Zealand, seeking to inspire others with his video and manifesto, just as he had been inspired by such content. This incident — and particularly social media’s role in fueling real-life harm — drove policymakers and technologists to try to block online terrorist and violent extremist content, or TVEC. Since then, there’s been unprecedented progress and unity among governments and platforms toward quelling the spread of TVEC, from the ambitious multilateral Christchurch Call to Eliminate Terrorist and Violent Extremist Content Online, spearheaded by former New Zealand Prime Minister Jacinda Ardern and French President Emmanuel Macron, to the technology industry’s Global Internet Forum to Counter Terrorism crisis response mechanism.
Yet the problem persists. In fact, it will become more challenging as media manipulation tools such as deep fakes and sock puppets grow in sophistication. So what are policymakers globally missing in the fight against such abhorrent material? And what are the practices tech companies need to put in place now to more effectively tamp down on its rapid spread? The answers can be found both in technological solutions but also a collective willingness to accept that mistakes will happen in service of swift removal of content that makes the internet deadly.
Critics who say there isn’t enough happening to take down TVEC usually point fingers at companies’ entrenched interests or policymakers’ limited technological know-how. Beyond these often-discussed issues, we highlight in a recent paper two critical, yet underappreciated barriers to addressing TVEC that deserve greater attention in our quest to make the web safer.
First, our most scalable option for policing social media — machine learning tools — is inherently imperfect. Second, our inability to even define or agree on what is meant by terrorist or violent extremist content has narrowed our scope of coverage to give neo-Nazi, white supremacist and violent misogynistic content a free pass.
On the first problem, the vast scale of social media presents a myriad of technical problems. Meta estimates that it gets more than 1 billion postings per day. YouTube gets around 3.7 million new videos per 24 hours. Manually policing this content would require approximately 34,000 human reviewers for YouTube and 1.25 million for Meta. The only possible solution requires that the process of review be automated. However, current approaches come with a host of problems.
A primary method used by the tech industry is hash matching: using a hash (or digital fingerprint) of known terrorist content to identify identical or near-identical posts. This is aided by a library of TVEC hashes kept by the Global Internet Forum to Counter Terrorism (GIFCT), an industry consortium established in 2017 by Twitter, Microsoft, Meta and YouTube. It has improved crisis response. After the 2022 shooting in Buffalo, for instance, tech companies’ responses were much faster compared to the response after Christchurch. Even so, one video on Streamable was viewed 3 million times.
Such filtering is inherently reactive and can only discover reposted TVEC content, not nip the problem in the bud by flagging original posts. Finding TVEC hashes in the first place is often a slow manual process. Recognizing new content (or slight alterations of existing content) requires some form of AI, likely in the form of machine learning. This involves training an algorithm on known examples of TVEC so that it can pick out and block similar content.
The limitation of this approach is that it is inherently statistical in nature. This means that the machine must decide on a probability of some content being an instance of TVEC. Inevitably, some content will be miscategorized — genuinely TVEC content that is seen as benign (a false negative) or content that is not TVEC but looks enough like it to the algorithm to be blocked (false positives).
This leads to predictable but unfortunate results. False positives lead to claims that platforms are suppressing content that, for example, documents war crimes. False negatives mean that some content from these groups will make it on to the platform. Like attempts to eliminate spam email, these statistical approaches will not be perfect, but they can and should improve. With an ideal goal in mind, we can train detection tools to become mostly fair, accurate and scalable.
While these technical problems are significant, far worse is the political problem of agreeing on just what constitutes TVEC. There is little or no agreement on what constitutes TVEC. As shown by the recent injunction against the U.S. government even talking to social media companies about “constitutionally protected free speech” with no clear guidance of how to distinguish that speech from anything else, determining just what is meant by TVEC is difficult and contentious.
Today, most large social media companies advertise that they automatically remove 95% of TVEC. But their conception of “TVEC” might be much narrower than assumed – a more inclusive definition could increase the number removed, and the number failed to be removed, by orders of magnitude, and radically change the percentage of content automatically removed. Without a common notion of what constitutes TVEC, calculations of percentage removed are meaningless.
The closest thing we have to an international organization trying to establish a taxonomy around TVEC is the GIFCT, which overwhelmingly focuses on lowest-common-denominator groups that are agreed upon at the UN to be terrorist organizations, such as ISIS and other Islamic terrorist groups. As a result, many organizations that embrace violence and terror — especially neo-Nazi, white supremacist, and incel communities — often escape strict scrutiny.
More troubling, such narrow conceptions of TVEC center on the organizations that generate the content, rather than the content itself. Many examples of TVEC are created and posted by lone-wolf individuals with no known connection to terrorist groups — the Christchurch shooter, for one. Further, it is what these people say and do, not merely their affiliations, that matters.Taking down posts based on content can quickly run afoul of free-speech guarantees. But manifestos and live-streams like those posted by the Christchurch shooter are not going to be identified by the originating organization. If we wish to keep these from the internet, content-based moderation will be needed.
Characterizing and restricting TVEC based on substance, not organization, requires subtlety, and must take care to not impinge on free expression. This dynamic between free expression and safety is a balancing act that we have been attempting for hundreds of years; the fact that it is difficult should not preclude us from attempting to find a better balance for society.
If we wish to make progress in our quest to remove TVEC from social media, we would do well to redirect our efforts with these difficulties in mind. More than simply identifying organizations whose content should be removed, we should concentrate on refining tools to characterize the content of TVEC. We should hold technology companies to high standards but expect improvement rather than perfection in detection and removal of this content, knowing that there will be mistakes along the way.
We should not allow “perfect” to be the enemy of “better,” and we should be ambitious yet set our expectations appropriately. This is a complex issue, not a battle that will be won by a single advance in either the policy that characterizes TVEC or the technology that flags and removes it. But this is a battle that we can win over time, making things a bit better every day.
Gabrielle (Gabe) Armstrong-Scott was a Graham T. Allison, Jr. Fellow at Harvard Kennedy School’s Belfer Center for Science and International Affairs.
Jim Waldo is the Gordon McKay Professor of the Practice of Computer Science and CTO in the School of Engineering and Applied Sciences at Harvard, and a Professor of Policy teaching on topics of technology and policy at Harvard Kennedy School. Prior to Harvard Jim spent over three decades in private industry, much of that at Sun Microsystems.
Updated July 20, 2023: The original version was updated to correctly identify the city of Glendale, Ariz.